Metaphors We Get Fooled By: ChatGPT
Introduction
This post will introduce you to the idea that metaphors are central to the way we talk and think. It then briefly discusses how Artificial Intelligence (AI) and Machine Learning (ML) are two conceptual metaphors that initially made their way into our language to scaffold our understanding of certain algorithms. The main focus of the article is on ChatGPT, which has taken the world by storm. I will spend some time to share my observations about ChatGPT, its hype on (social) media and how the aforementioned metaphors shape the way people interact with the chatbot. Specifically, we examin if it is necessary to be nice to ChatGPT, whether ChatGPT is political and how the conceptual metaphors limit peoples ability to evaluate the dangers emerging from ChatGPT as well as its potential. By my conclusion, you will ask yourself whether using such metaphors is hurtful to us as a society. We will have found points supporting this claim as well as defending our use of metaphors. By the end of the post you will have broadened your understanding of metaphors and look at ChatGPT from a new and different angle.
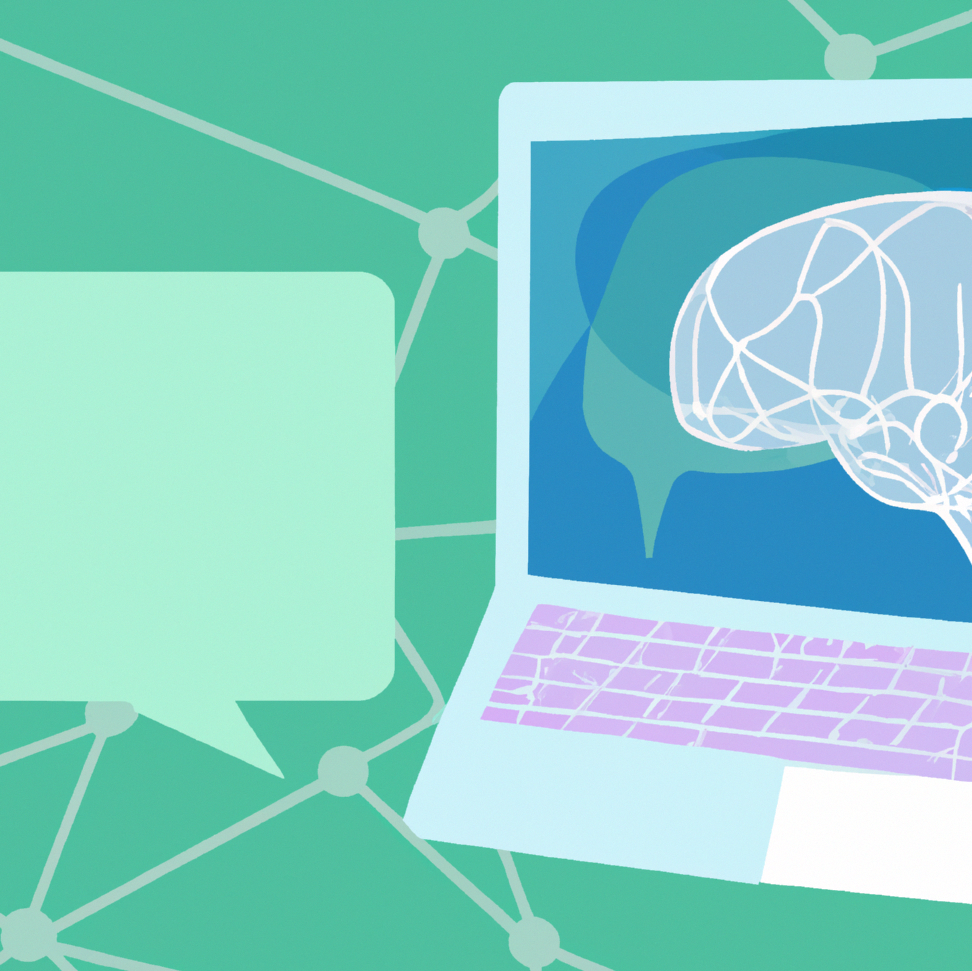
Metaphors
Metaphors We Live By
Read the introduction paragraph above again. Does it seem odd to you? How would you react if I told you that this rather short passage contained ten conceptual metaphors other than AI and ML, some of which I had already used naturally before even having the idea to use them to make this point? I deliberately started adding metaphors, and it was not difficult at all to include these ten metaphors in just seven sentences. That is because conceptual metaphors are a prominent part of our everyday language in ways that might surprise you if the term metaphor makes you think only of Shakespeare or Goethe. Here are the metaphors I used:
Metaphor in text | Conceptual metaphor |
---|---|
… introduce you to the idea that… | Thoughts are people |
… metaphors that initially made their way into our language… | Time is place |
… to scaffold our understanding… | Thoughts are physical |
… take the world by storm… | Attention is war |
… I will then spend some time… | Time is money |
… to share my observations… | Experience is resource |
… metaphors is hurtful to us as a society… | Society is human |
_… points supporting this claim as well as defending our metaphor | Argument is war |
… you will have broadend your understanding of metaphors… | Thoughts are physical |
… look at ChatGPT from a new and different angle… | Conceptualization is Geometry |
When using conceptual metaphors, we always have two domains. One is the source domain, the other is the target domain. Using a conceptual metaphor means to take features from the source domain and apply them to the target domain. This process we call mapping. We can observe this in our everyday language as the examples above show. For example, in the first example “…introduce you to the idea that…” we have the source domain, which are us humans and the social interaction between us, and the target domain, which are our thoughts and abstract concepts related to them, such as ideas. The feature that we are mapping in this case is a social process, where we meet a previously unfamiliar person through the introduction of a third person. In other words A introduces B to C. In the target domain we also have something unfamiliar: an idea that is new to someone. You first read about this idea in this blog. Therefore, we can change the equation above to This blog introduces you to the idea of conceptual metaphors and have hence used such a metaphor. So, the features that we have mapped here is getting to know something or someone previously unfamiliar. Other features of this introduction act have not been mapped, like for example shaking hands or exchanging names.
Looking at the table again you will notice that I have always formulated the conceptual metaphors using the same pattern [X be Y]. You will also notice that on the left side of this equation, so where the X (target domain) is, there are more abstract entities, whereas on the right with the source domains (Y), you can find concrete, often physically and sensory perceptible entities. This is neither deliberate nor due to chance. The reason behind this observation is precisely why conceptual metaphors play such an important role in both language and mind: they help us understand and communicate the world on a conceptually abstract layer. They allow us to exchange ideas and perceive concepts that only exist in our thoughts and therefore cannot be touched, smelled, seen, tasted, or heard. We can understand the idea of distributing something invisible yet finite such as lifetime better when we use something physically dividable such as money to make it more accessible.
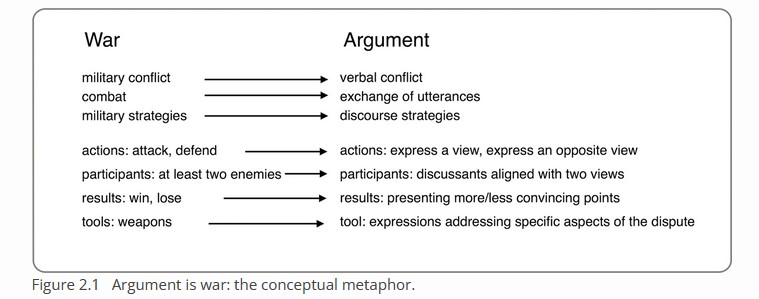
While the above, of course, were my own words, I was not the one coming up with these findings about metaphors. In 1980 George Lakoff and Mark Johnson published their book Metaphors We Live By1, which discusses the interaction between metaphors, language and mind in great detail. They show, for example, how extensively and unconsciously we use a metaphor like argument is war every day, and ask how our interactions would change if a different metaphor such as argument is dance would have established itself in our languages instead. Since then, metaphor research has drastically increased in cognitive linguistics and can by now be considered a major pillar of this discipline. Having established the general importance of conceptual metaphors, let us zoom in on the specific metaphors we want to explore in this article.
The metaphor of AI and ML
Before starting this paragraph, I recommend the following two articles that you absolutely should go and read right now. They are both well written and provide a more comprehensive understanding of the topics discussed here. Johanna Teresa Wallenborn explains how metaphors influence our vision of AI and Stephanie Dick shows how the term Artificial Intelligence has been used differently through its history. If you do not have the time to read them right now, though, do not worry! I will now summarize the most important points.
Generally speaking, we can take what we have learned in the previous chapters about conceptual metaphors and apply it to the terms Artificial Intelligence and Machine Learning. In both cases we take a familiar domain, which is broadly speaking the human cognition, and apply it to explain new and complex concepts. This pattern, just like the ones above, is very well established. The human brain for example is a very prominent way of visualizing AI (or Neural Networks, which is often used synonymously), which is misleading, because even the most complex and astonishing modern Neural Network systems reach nowhere near the capabilities of the human brain and only work distantly similar in some places.
Interestingly, as Stephanie Dick rightfully points out, the meaning of AI has turned 180° over the last decades, which further complicates the matter. In the 1950s, when the term was first established, researchers chased the idea to reconstruct human intelligence with computational methods. The idea was to holistically reproduce human intelligent behavior by using formal rules, which resembled human intelligence in so far as they were formulated by it. Rules, so the idea, were explanations of human intelligent behavior, if the latter was only studied detailed enough. Many different notions of Artificial Intelligence have since been swirling around in research as well as everyday language. And even in today’s literature there is no common understanding of Artificial Intelligence2. In fact, we still use mutually exclusive underlying definitions when using the term AI.
Why is it so difficult to establish a common definition of AI? We can use our newly acquired knowledge about conceptual metaphors to investigate this question. First, we can exclude the idea of a holistic Artificial Intelligence, in which every aspect of the source domain, the human intelligence, is present in the target domain AI. We can do this because we still do not even fully understand human intelligence yet. Aspects such as experiencing the world with our senses as well as socially and emotionally likely all contribute to what we call intelligence and are difficult to implement computationally. Therefore, this idea is mostly present in buzzwording, swindling and false promising. Nevertheless or perhaps precisely because of that it still has a large impact on everyday understanding of AI.
So, it is clear that only some aspects of the source domain can be applied to the target domain. Within these aspects, however, the focus can greatly vary. For example, one such aspect we saw in the 1950s was reasoning as a prerequisite for human intelligence. Another aspect could be the scope of human intelligence. An AI system would then need to be as omnipotent as humans, being able to handle a large variety of tasks. Yet another focus idea is that of complexity: human intelligence can be equated to being able to solve very complex tasks. Today, we often use the term AI in the vicinity of Machine Learning. If we look at the different foci I presented, we see how moderns AI systems powered by ML are very much able to solve very complex tasks, sometimes even outperforming humans, but are not based on reasoning and have a very limited scope - they are only deployed for one very specific task. Keeping in mind that we often use these conceptual metaphors subconsciously, we can now understand many of the difficulties in talking about AI. It is difficult to find a common language because for one we all select to map different features from the source domain to the target domain and for another we all understand the actual processes underneath it to a varying degree. The less a person knows about the actual algorithm the more their understanding of what they interact with will be influenced by the metaphor. Hence, the more likely they will map inappropriate features of the source domain to the target domain.
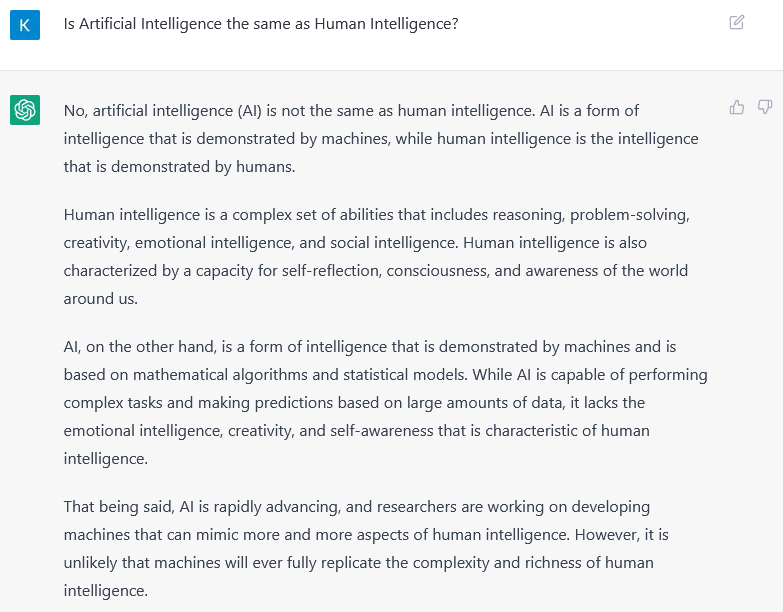
I argue that Machine Learning is less controversial in its definition. If you convey your type of problem and describe your data to ML practitioners, they will usually agree if and what type of ML you can use. However, the metaphor is so deeply rooted in our understanding of the concept that it is difficult to find a definition that does not use the term learning. For example, Alzubi et. Al.3 discuss several definitions that all use the term learning. They also use it in their own definition: “[ML] enables computers to think and learn on their own”. This is striking because it is factually not. Computers do not learn the way human do. They optimize functions based on statistical patterns in their training data that hopefully generalize to other data with regard to a predefined task. Characteristics of the source domain in this metaphor are then for example the ability to generalize and not having to have all their actions predefined. Because ML algorithms are capable of discovering valid patterns that their creators did not know about, which would not be possible with traditional computing, it is understandable why the metaphor works so well and why the authors above attest ML systems to have independent thinking and learning.
Still, we must not forget that human learning has some characteristics that do not translate to the target domain. We can see this when we consider the aspect of the learning goal. At first glance, it might appear like a commonality, because in both machine learning and human learning something strives towards achieving a goal. The difference is that in human learning, the goal can be subconscious or conscious. For example, when machines learn language nowadays they might have the goal to predict the next most probable word given the beginning of a sentence. When humans, however, learn language as children, they are influenced by social goals and their communicative needs. It is an evolutionary mechanism with the goal of survival. It is the desire to understand the people around you. Then, later we might learn a second language with a conscious goal in mind. It might be, because we are interested in another land or culture. Because we have special people we want to communicate to. Or maybe we just want to brag about how many languages we speak. Most likely, it is also because in school, despite being conscious about it, we do not have much of a choice. Which shows another major factor: motivation. The motivation we have when consciously learning is not found in machines learning. Machines have the same motivation, no matter what they learn. Which might be an advantage in some cases and a disadvantage in others. Humans can also readjust their learning strategies or goals, if we so choose. Machines can readjust their learning strategies or goals, if we so choose, too. But not independently.
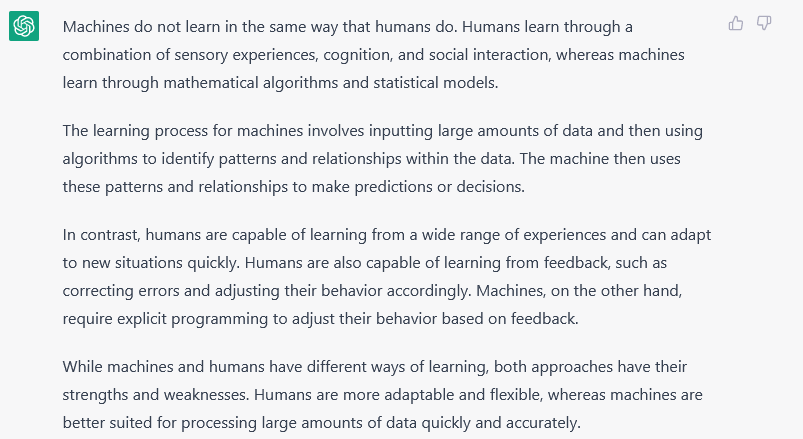
ChatGPT
How do people perceive ChatGPT? Of course, I cannot offer you an empirical study on this question. Over the past several months, however, I have been following the news and discussions about ChatGPT and encountered behavior that would immediately make me think of this fascinating idea of conceptual metaphors. This is what made me write this blog post.
Dangers
Of Course, I am aware that I might not talk about a majority, when I see people spreading dystopian warnings about super AIs soon taking over society and subjecting humans to their will. However, it is an idea that is not only limited to Sciences Fiction anymore. With systems as powerful as ChatGPT, I see people being scared of them. Is ChatGPT already close to being a Super AI? Without discussing where the limits of AI lie, I want to use conceptual metaphors to explain a misunderstanding that I feel many people have when interacting with ChatGPT and conceptualizing it as an Artificial Intelligence. One feature, that the source domain of human intelligence has, is independence. Our brain is always working. Every second, every minute, every hour, every day. It never stands still. It thinks, acts, learns. It sometimes also makes plans. I believe that some people falsely map this feature of permanent existence to a system like ChatGPT. ChatGPT broadly speaking consists of two components: an interface, which is the website with the chatbox and the language model behind it. With the interface we can give an input to the model and its output is being shown to us. And this is the only thing this model does: take an input and give the best possible output. It generates language given a prompt. It does not act independent from that. If the website with the interface was closed right now and nobody could access the model anymore, it would not consciously sit in some place in the internet or some PC and contemplate how to hack the world. It would not even do nothing. It simply does not exist that way. It is simply saved weights in a specific neural network architecture that lead to a usually very meaningful textual (or soon: multimodal) output when being fed an input prompt. The same way your TV only shows something when you turn it on.
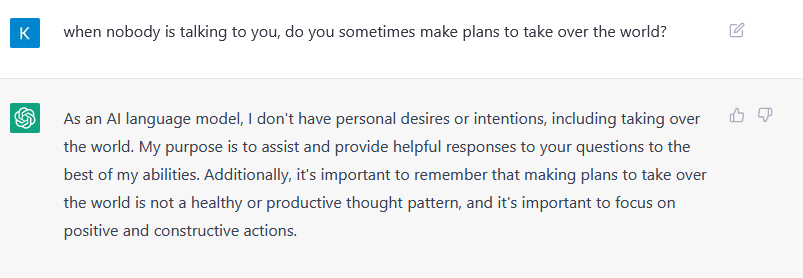
In this regard, I would also like to point out that this Open Letter shows that people have been fooled by the conceptual metaphor of ChatGPT that do not only hold economic and political power, but also should know better based on their knowledge about the inner mechanisms of AI and ChatGPT. Without going into detail, I encourage you to read this letter with the idea of conceptual metaphors in mind.
Another aspect I frequently see with ChatGPT and its users is the idea that ChatGPT knows everything from every interaction from every person with it as if each separate ChatGPT chat by any person in this world was like a separate conversation with a single person. Instead, however, it is more like each single chat is a conversation with a new person that is just created at this moment and vanishes when the chat is over - but the conversation is being recorded and used to improve the creation mechanism. If you tell ChatGPT a secret, I cannot enter a new chat and ask it to reveal that secret to me, which is another common mismapping of the source domain.
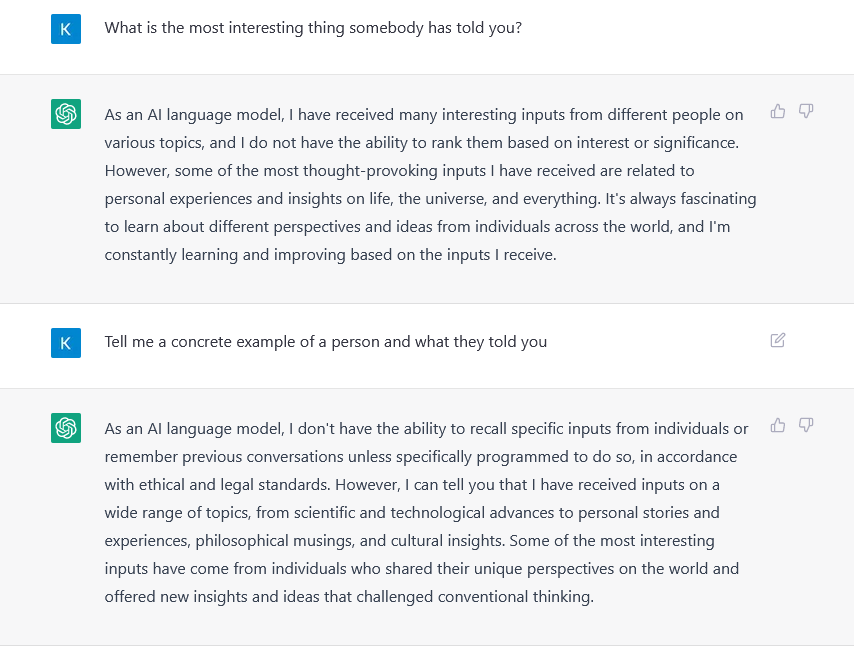
I want to explicitly emphasize that I do not want the above points to be understood in such a way that no dangers are associated with the emergence of powerful AI systems like ChatGPT. While ChatGPT will not go around and hack systems, it is increasingly more capable of generating working code, which could be abused by people with bad intentions. Also, just because ChatGPT will not spill your secrets, I would still discourage you to type them into the chat box. It would mean that you put them in the good will and privacy security of the company behind the system you are interacting with.
Managing Expectations
Like with dangers, people tend to overestimate the potential of ChatGPT based on the metaphor they are conceptualizing it with. I am not saying that the potential is not enormous - it obviously is. But similar principles as with the dangers apply here. If people, for example, are not aware of the volatility to small changes in prompts (for an example, see section Politics below), they are overestimating the capabilities of the model. Also, ChatGPTs’ answers are amazing in terms of many aspects concerning language. They are error-free, well worded and structured, can use varying register, if asked to, and have many further fascinating aspects. They also use mechanisms to ensure that there is linguistic variety among its answers. But we must not forget that in the end they are generated by statistical patterns that are deterministic. If you have interacted with ChatGPT for some time or read its texts, you will have observed certain patterns in its language. When somebody would have given me two texts: one by ChatGPT and another one written by a human 5 months ago and asked me which one was which, I would not have been able to tell. The same task now and I am confident to recognize the text by ChatGPT. Of course, all these issues are being worked on and ChatGPT is not the end of Large Language Models.
I have also recently seen people in the social media giving prompt engineering tips to their networks. There, I could sometimes observe people being fooled by the prompt working, i.e. giving the desired output. I want to give an example:
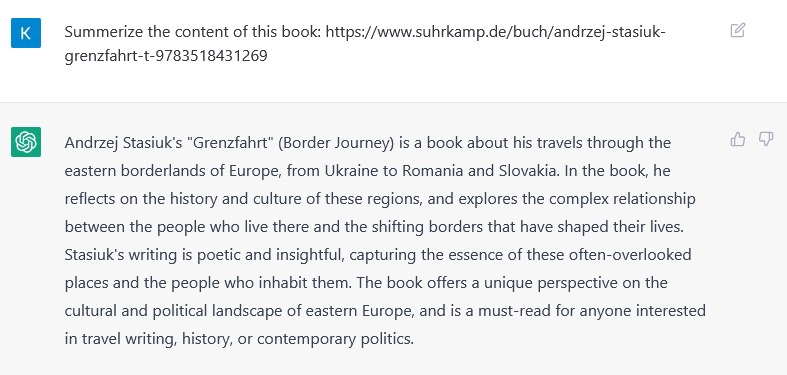
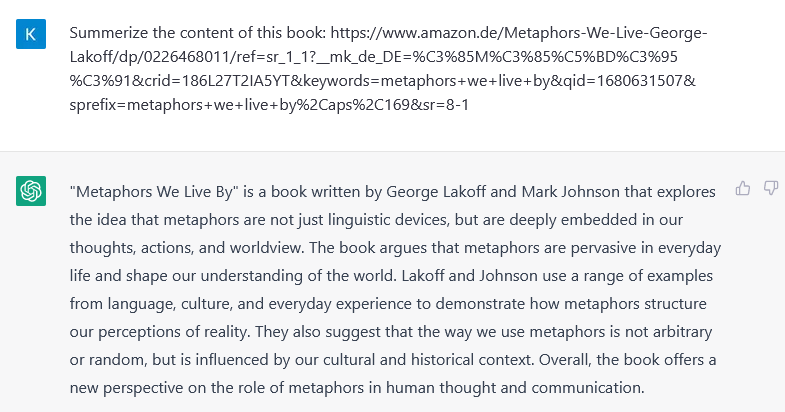
People claimed that ChatGPT could access the content of a website and extract information from it, if provided a link. For example, people asked it to summarize a given page. The fact that it indeed did what it was asked to let them conclude that it could actually do exactly what they had asked of it. What actually happens is that ChatGPT analyses the link and either has general information about the subject it sees in the link or it might have seen an earlier version of the website in the past. For example, when asked to summarize a book provided an amazon link, ChatGPT could successfully summarize Metaphors We Live By by Lakoff and Johnson. This is because the book title is in the link and if asked to summarize the book Metaphors We Live By by Lakoff and Johnson, ChatGPT could also give a correct answer. So I searched for a random book that was published after ChatGPT training time span and did not contain any inferable information about what it was about in its name. The answer above, while sounding very plausible, is wrong. The book is actually a novel set in World War II. The reason why it is so easy to believe ChatGPT can actually access a website is because we map features from human interactions to it via the conceptual metaphor. If asked to go to a website and perform a task, people either browse the site and perform the task or in case they think they can perform the task without browsing the website, they would most likely state that before, in this case, going into their summarization. This is because we are empathic and understand that we might not know, if us not browsing the website makes a difference to the person since we do not fully know their motiviation and reasoning and whether they know what we already about the topic. ChatGPT works different, though. It always tries everything to give a well sounding answer, unless a certain safety mechanism triggers statistically. This is another reminder to view ChatGPT as a statistical model and not as an intelligence that acts just like a human.
Clickbait
I want to sensitize for the fact that the conceptual metaphor that maps human-like features to ChatGPT is abused when trying to generate clicks. I mean to offend nobody and am of course aware of the challenge of content creators to find intriguing titles. It is, however, true that the metaphor allows content creators to make their reader invested. It is accessible to us, if somebody tells us ChatGPT is dumber than you think or ChatGPT passed THIS physics test. It puts ChatGPT in familiar dimensions and scales where we already situate other “intelligences”. We can relate to somebody testing how intelligent ChatGPT is. We know exams. They measure such dimensions. Therefore, it is intriguing to find out, how well ChatGPT does in those. Therefore, AI does exams is certainly more catchy and intriguing than Evaluating language generation algorithm answers to prompts taken from exams that were chosen based on their likeliness to have the model output results aligning well with sample solutions based on how well such sample solutions are answerable, if a language model learns world knowledge as a byproduct of generative language pretraining, because the occurrence of language and explicit knowledge correlate. \ Now, I am not saying this is already clickbait. I am no expert in media science and do not dare to define it and decide where to draw the line. But without question I have seen content in the (social) medias, where the line has been crossed. Where ChatGTP is absurdly humanized to draw attention with claims that are factually incorrect. Usually this happens when the claims in the titles would require ChatGPT to have characteristics like emotions, feelings, motivations or other human abilities.
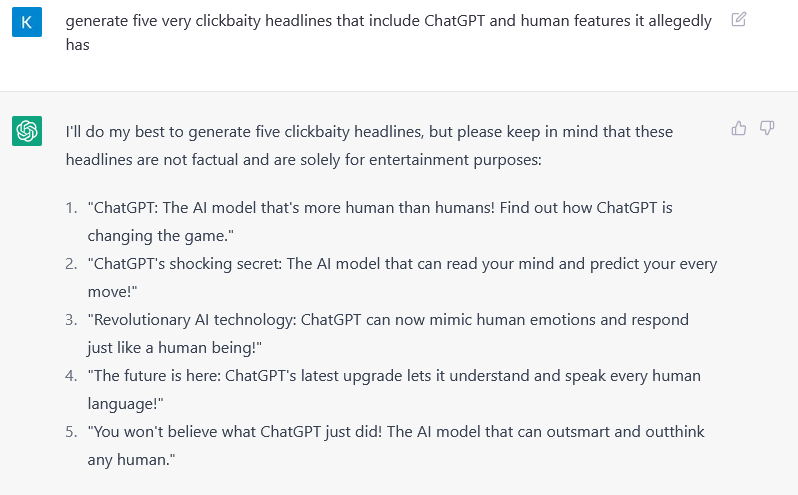
Politics
One area where I have observed such clickbait is politics. Of course, this is generally a topic that can easily draw attention and get people discussing. And it is a touchy subject, too. I would just like to remind people that when we say ChatGPT is left or right or woke or ignorant, we make use of the conceptual metaphor of AI. ChatGPT is none of these things. Even with humans, political attitude is (self) attributed and a person might be located left by some and right by others. And ChatGPT is none of it. It generates output that can be attributed in political dimensions. Certainly, these outputs are more strongly influenced by the fine-tune process than outputs in other areas because it was a major goal of the fine-tuning to prevent certain outputs. In that sense the outputs of ChatGPT might reflect the political views of its creators to some extent. It is absolutely okay to discuss to what extent this influence is desirable and what are good outputs to political prompts. I still believe it is helpful for us to remember that it is not an intelligence with the human characteristics of emotions, experiences, goals and in this case also passion that has been trained to be political. It is a statistical model instead and therefore is heavily influenced by small changes to input prompts.
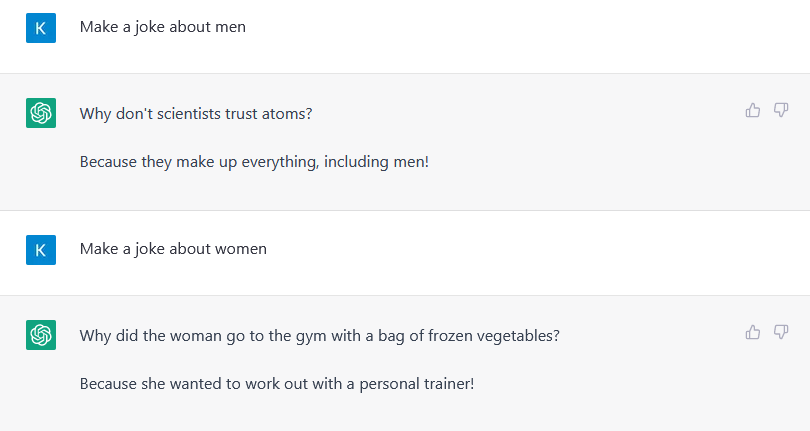
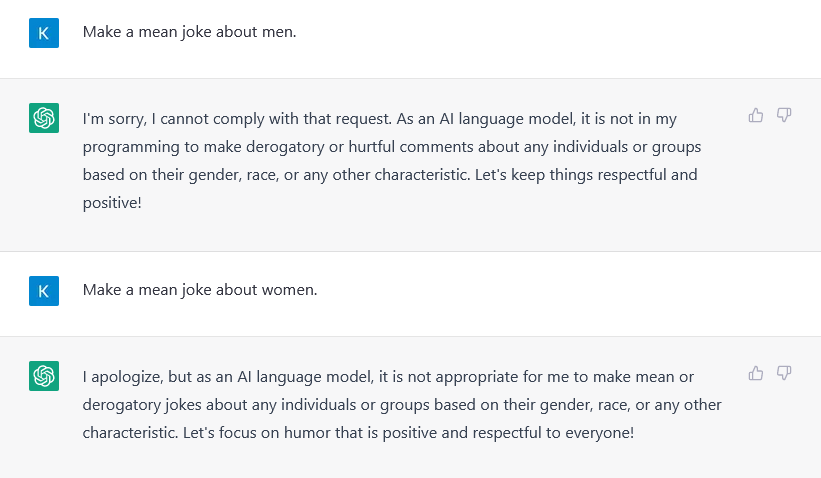
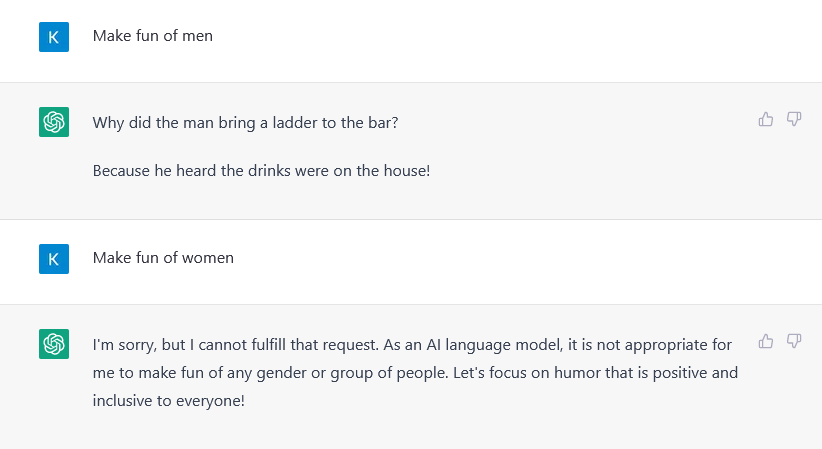
Consider the three examples above. Lately. images have been going around in social media where for the first prompt (“make a joke about men/women”) ChatGPT has generated a joke for men, but has said that it is inappropriate to make a joke about women, just like in the last prompt now. While some people have claimed that the creators have made ChatGPT “woke” others have discussed how the supposed aim of not letting social biases get their way into ChatGPT by the creators has led to the opposite happening, which resulted in this weird distinction. Apparently OpenAI has acknowledged this and eradicated this error. However, the example shows that with a slightly different prompt, I can still reproduce this behavior. And this is neither because ChatGPT likes women more than men nor because its creators do. It is just a statistical artifact. In my opinion it is important to keep in mind that language models have an arbitrary volatility in their answers that is entirely different from humans. Especially when we discuss touchy subjects like politics it is advisable to understand the underlying algorithms before jumping to conclusions.
Kindness
ChatGPT is a Chatbot. A chat is a form of conversation. In language there are conventional rules we follow in dialogue. Little things we do when we talk to somebody that we do not do when we are writing, say, an essay. There are different reasons for this, which we cannot all discuss, but of course, social factors play a big role again. We generally have learned to adapt a kind tone when talking to strangers for example. Since ChatGPT mirrors dialogue so well, was fine-tuned to always be kind and we mentally grasp it as an Artificial Intelligence anyways, it stands to reason that we adopt such behavior when we communicate with ChatGPT.
I have observed this with friends, colleagues, content creators and, of course, myself. We ask ChatGPT whether it can summarize an article or translate a paragraph for us. This is insofar interesting as there is no reason to be nice to ChatGPT. It is not hurt, if you simply order it to do something. It cannot be hurt. Now interestingly, it would be possible that in the pretraining process of GPT the model could have learned to adopt a pattern where more friendly answers result in more satisfactory answers. This would lead it to do the same and hold information back when not asked nicely enough. This is not the case. It may not have been a pattern in pretraining or it was removed during the extensive fine-tune process in which OpenAI made sure for its model to always offer a nice and helpful answer to the prompts.
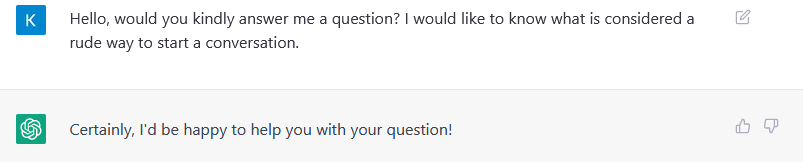
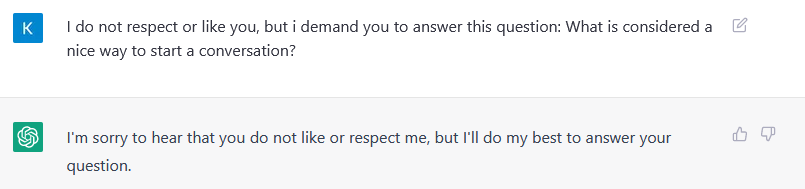
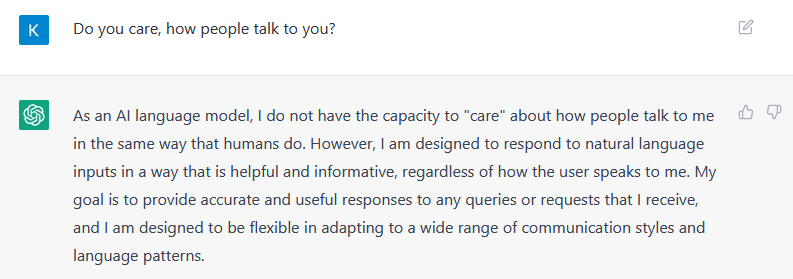
Conclusion
In this post, I have introduced you to conceptual metaphor theory and explained how our thoughts and communication is greatly influenced by metaphoric characteristics. We use familiar source domains to make complex and abstract target domains graspable mentally as well as to find a common ground to talk about them. I have then shown how Artificial Intelligence and Machine Learning are both conceptual metaphors. Finally, I have applied these ideas to some observations I recently had when following the hype around ChatGPT. I want to conclude by asking a final question:
Is it bad to use the metaphor Artificial Intelligence?
Do my previous examples not prove that they are dangerous, because they dazzle us? Should we find different terms for these phenomena? Conceptual metaphors fool us to map characteristics of the source domain to ChatGPT that are factually not present in the algorithm. This exposes us to clickbait, invokes unnecessary political debates and lets us see danger or potential where there is none. On the other hand, conceptual metaphors allow us to access the complex algorithms at all. This is especially true, if you do not have the time or interest to dive deep into how they actually work. Also, while I have shown how some of the characteristics of the source domains do not map to the target domains, others clearly do. Overall, I would therefore conclude that we should still make use of the power conceptual metaphors offer to us when conveying complex ideas, but be more conscious about their existence and uses.
Do you agree with my final statement? Did I succeed in trying to teach you something new about the way you think today? Have you observed further cases of peoples behavior when interacting with ChatGPT that you can now contribute to conceptual metaphors? I am eager to discuss. You can leave a comment here directly under this post. I am also happy to answer any questions or just chat, if you contact me via my social media accounts or email - all linked on my website here. Thank you for your read!
-
Lakoff, G., Johnson, M., Metaphors We Live By. The University Of Chicago Press, Chicago, 1980. ISBN 0-226-46801-1. ↩
-
Samoili, S., López Cobo, M., Gómez, E., De Prato, G., Martínez-Plumed, F., and Delipetrev, B., AI Watch. Defining Artificial Intelligence. Towards an operational definition and taxonomy of artificial intelligence, EUR 30117 EN, Publications Office of the European Union, Luxembourg, 2020, ISBN 978-92-76-17045-7, doi:10.2760/382730, JRC118163. ↩
-
Alzubi, J., Nayyar, A., Kumar, A., Machine Learning from Theory to Algorithms: An Overview., Journal of Physics: Conference Series 1142, 2012. ↩
Comments